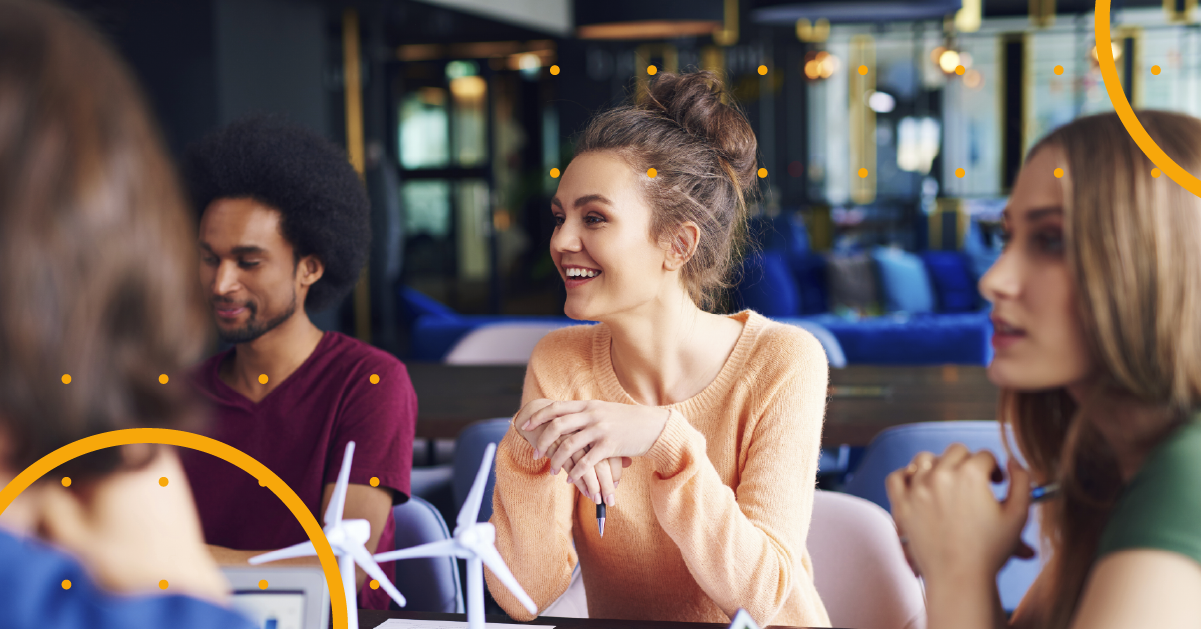
Introduction
Artificial Intelligence (AI), with its boundless potential and creative methodologies, is poised to play a pivotal role in the battle against climate change. By optimising energy usage, strengthening resilience to climatic adversities, and driving the reduction of greenhouse gas emissions, the influence of AI in climate action is progressively coming to the fore. Research spearheaded by the esteemed Capgemini Research Institute paints an encouraging picture, envisaging a formidable 16% reduction in greenhouse gas emissions over the next three to five years, as a result of AI applications. However, it is essential to bear in mind that AI also presents substantial environmental challenges. Large-scale AI models necessitate significant computational resources, thereby consuming vast quantities of electricity, often sourced from non-renewable means. Thus, it is imperative to strike a balance between the benefits and drawbacks of AI in mitigating and adapting to climate change, and to institute sustainable practices and policies for AI development and application.
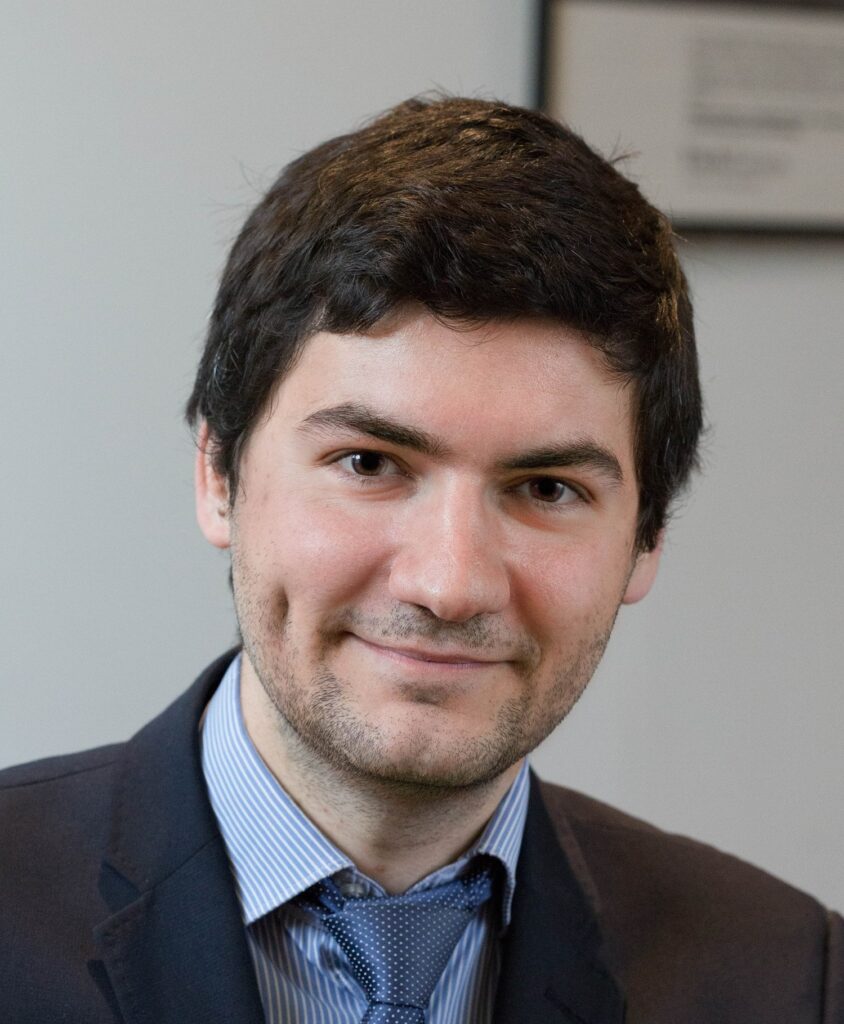
Dejan Glavas is an Associate Professor of Finance at ESSCA School of Management (France). He holds a Ph.D. in sustainable finance from ESCP Business School and has published his work in academic journals such as the Journal of Cleaner Production, Finance and Bankers, Markets and Investors. His work specifically focuses on ESG, Artificial Intelligence, Business Valuation and Green Finance.
The Power of AI: Augmenting Energy Efficiency and Reducing Emissions
AI provides vast opportunities for bolstering energy efficiency and fortifying our collective ability to navigate the complex challenges posed by climate change. A comprehensive study conducted collaboratively by PwC and Microsoft illuminates the capacity of AI to curtail greenhouse gas emissions in the energy sector by 1.6% to 2.2% by 2030, relative to a baseline scenario.
This promising scenario is brought to life through a significant collaboration between DeepMind and Google. Their joint venture has led to the successful application of machine learning algorithms in a 700-megawatt wind farm in the United States. By employing a neural network that was trained on a plethora of weather forecasts and historical wind turbine operational data, DeepMind has configured its system to accurately predict wind energy production up to 36 hours in advance. These sophisticated predictions enable the model to offer recommendations for optimising electricity delivery commitments to the power grid a day in advance. Consequently, the implementation of machine learning has boosted the value of the wind energy generated by the wind farm by a substantial 20%.
AI as a Data Integrator: Building Resilience Against Climate Change
AI emerges as a formidable weapon in enhancing resilience to climate-related hazards. Resilience is defined as a system’s capability to foresee, withstand, adapt to, and recover from the impacts of a hazardous event. AI demonstrates the unique ability to amalgamate data from an array of sources, such as satellite remote sensing, ground-based sensors and hydrological models. This ability endows AI with the capability to map regions that are susceptible to environmental adversities like floods, droughts, and landslides.
Furthermore, AI can facilitate the development of early-warning systems and predictive modelling, thus optimising response times and guiding critical decisions. Hence, by exploiting AI, we can diminish our carbon footprint and adapt effectively to the repercussions of climate change, which are anticipated to affect billions of individuals residing in high-risk areas.
The ‘Red AI’ Dilemma: The Environmental Footprint of AI
While the potential of AI in battling climate change is substantial, it is crucial to acknowledge the environmental impact of AI. This primarily stems from its significant energy consumption, a characteristic often referred to as “Red AI”. A study conducted by the University of Massachusetts reveals that the carbon footprint generated by training a single natural language processing model can be nearly three hundred tons of CO₂ equivalent. This carbon footprint is equivalent to 125 round-trip flights between New York and Beijing or five times the lifetime emissions of an average car.
As the performance of AI improves, it necessitates the expansion of databases, resulting in a marked increase in greenhouse gas emissions. For example, the design and training of translation engines can generate up to 280 tons of CO₂. Additionally, data centers and high-performance computing facilities contribute around one hundred megatons of CO₂ emissions annually, akin to the emissions from American commercial aviation.
Moving Towards Sustainable Solutions: Mitigating the Environmental Impact of AI
A range of potential solutions are available to address the environmental footprint of AI. These encompass powering data centers with renewable energy, optimising algorithms and architectures and fostering the sharing and reusing of pre-trained models.
According to a report from Vertiv, data center operators are fervently intensifying their efforts to incorporate renewable energy strategies. Renewable energy sources, energy storage systems, and lithium-ion batteries play an instrumental role in delivering sustainable and dependable power to data centers. Moreover, data centers are increasingly exploring water-free cooling solutions and progressively replacing high Global Warming Potential (GWP) refrigerants with alternatives that have a lower GWP.
One vital approach to reducing the carbon footprint of AI involves deploying less energy-intensive algorithms. A research study comparing the energy efficiency of commonly used machine learning algorithms found that the Extreme Gradient Boosting (XGBoost) algorithm emerged as the most efficient, considering accuracy, execution time, and energy efficiency combined.
Encouraging the sharing and reusing of pre-trained models is another viable strategy for mitigating the environmental impact of AI. Pre-trained models, trained on large-scale datasets, can be fine-tuned for specific tasks. Reusing these models reduces the need for repeated training, thus conserving energy and reducing emissions. A study suggests that reusing pre-trained models can cut the carbon footprint of natural language processing tasks by as much as 94%. Furthermore, sharing pre-trained models can stimulate collaboration and innovation among AI researchers and practitioners, and enhance the transparency and equity of AI systems.
Conclusion
Amid ongoing climate challenges, AI surfaces as a crucial ally in our transition towards a more sustainable future. By exploiting AI’s potential in enhancing energy efficiency, refining algorithms, and advocating for the use of renewable energy sources, we can strategically position AI as a potent tool in our fight against climate change. While AI poses environmental challenges, it offers a repertoire of powerful tools to address climate change and can assist us in making significant strides towards a more sustainable and climate-resilient world.