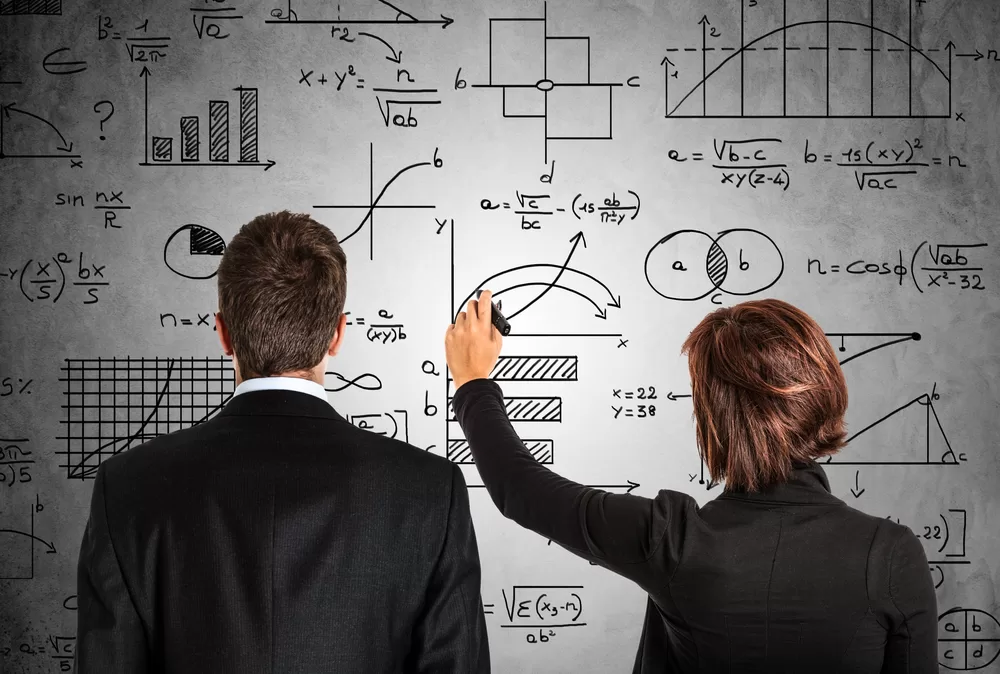
The objective of marketing has slowly shifted from product success to business success, and that’s a larger yardstick against which new age marketers are being measured. In one of the most challenging times for “marketing” as a domain, there seems to be some hope in what marketers avoided for a large part of their lives – logical & mathematical intel. A critical part of ‘marketing’ is user acquisition through demand generation. I don’t meet many marketers who understand the integration of these two factors, and at most times focus on ‘user acquisition’ without adequate demand generation. This is a sad but true sickness of marketers who are victims of practices that don’t define measurability correctly.
The following is an attempt to explain very simply the user acquisition process design along with demand generation, via a multi channel attribution system. At the heart of multi-channel attribution system lies the fact that users ‘engage’ with a brand or product across multiple channels, with just a probability of ‘action’ in any of those said channels. New age marketers like myself believe that it’s pointless measuring conversion from individual channels exclusively, when the true design of the system (along with the behavior) is built on a result generated due to the right integration of channels. This therefore means that most practices of current marketers are flawed and limited in scope.
The genesis of this model begins with customer “engagement” and the importance it has in the base definitions of this model.
A “true” or worthy engagement that can be counted in the demand numbers for a brand is defined by the “intent” of a customer for the brand at a given time “t”. A product of this intent and the target demographics defines engagement “E” for a brand at any given point in time. What we know for a fact is that this engagement is the only measurable and predictive factor that is available for us marketers. We know for a fact that customers with the right intent and demographics combination would engage in a specific channel, and that’s the best place for my brand communication to exist. Although, at that point in time of deciding to be available in a particular channel, all I know is that I am enabling “engagement” for my brand and creating demand.
The probability of engagement is a function of the time the customer will spend with the right “intent” inside that given channel. Any discovery, like my brand, would need to be intent based. Randomness (not serendipity) needs to be ruled out from the design. This means that more the time with the right intent, more is the probability of engagement with my brand, that I can establish through excellent discovery systems.
To explain this, I have taken an example of higher education marketing – which has matured/well defined phases in a consumer engagement process, although the channels of engagement are quite dynamic as per the candidates’ life cycles. In this example, I am considering the “research” and “prep” phases on the consumer/candidate side, which would mirror “discovery” and “networking” on the University’s side. The same can be applicable for any other consumer product/brand in other verticals/industries.
At any given point in time during the life cycle stages considered here, I am taking into account 6 different channels of engagement – Newspaper, Education Tours, Coaching/Prep institution, Online info portal, Publications, and College Visits. Let us consider that for any University brand, these are the 6 channels wherein candidate engagement can actually happen during the current life cycle stage. Given that there are possibly two outcomes – engagement and action, the number of combinations from which engagement and action can be generated are – 30 (Using 6P2 permutation)
Therefore the following would the possible combos of (e,a) which would “contribute” to the results OR customer acquisition:
M1 (E) | M2 (A) | M1 (E) | M2 (A) | M1 (E) | M2 (A) | M1 (E) | M2 (A) | M1 (E) | M2 (A) | M1 (E) | M2 (A) |
Newspaper | Edu Tours | Edu Tours | Newspaper | Coaching Inst | Publications | Publications | Newspaper | Online Info portal | Newspaper | College Visits | Newspaper |
Newspaper | Coaching Inst | Edu Tours | Coaching Inst | Coaching Inst | Online Info portal | Publications | Edu Tours | Online Info portal | Edu Tours | College Visits | Edu Tours |
Newspaper | Publications | Edu Tours | Publications | Coaching Inst | College Visits | Publications | Coaching Inst | Online Info portal | Coaching Inst | College Visits | Coaching Inst |
Newspaper | Online Info portal | Edu Tours | Online Info portal | Coaching Inst | Newspaper | Publications | Online Info portal | Online Info portal | Publications | College Visits | Publications |
Newspaper | College Visits | Edu Tours | College Visits | Coaching Inst | Edu Tours | Publications | College Visits | Online Info portal | College Visits | College Visits | Online Info portal |
Due to the fact that a candidate can engage at multiple places and take “action” in any of these places, it is only possible to calculate the probability of “Engagement in a channel” when there is a “Result” achieved in the system. This phenomenon can be calculated by taking each of the 30 sets (of channels) and running them through Bayes Theorem/Model and draw up a trend of the best possible result probabilities to design the marketing mix.
Step 1: Understand the “time with intent” spent for all the 6 channels, by the candidates-
[fusion_table]
Medium Names | Time Spent % age |
Newspaper | 0.1 |
Edu Tours | 0.2 |
Coaching Inst | 0.2 |
Publications | 0.1 |
Online Info Portfolio | 0.3 |
College Visits | 0.1 |
[/fusion_table]
The above table would also directly give us the probabilities of engagement across these channels for a given candidate at a given point in time. These would be used for further calculations and as input variables for the bayes model.
Step 2: Understand the “probability of result when there is a probability of engagement” in both the channels –
This is a key input given the fact that we are interested to understand what’s the probability of a result in a given channel considering there is a high probabilty of engagement in that given channel. This calculation includes input variables like – number of brand options at a given time in the channel, total time of engagement in hours over a time period, and the number of call-to-action from the brand’s side. Most marketers actually end measuring this and claiming to run ROMI on individual channels. As you can see now, that this is merely an input variable and not the final measurement.
Step 3: Run the numbers across all combinations and sort as per P(Channel|R) for each case –
If you run the multi probability Bayes model for each of the combinations and then sort the results as per the probability of engagement in a channel when there is a result in the system, you would see the following:
[fusion_table]
Medium 1 | Medium 2 | Medium 2 Score | Medium 1 | Medium 2 | Medium 2 Score |
Newspaper | Online Info portal | A++ | Edu Tours | Newspaper | B |
Edu Tours | Online Info portal | A++ | Coaching Inst | Newspaper | B |
Coaching Inst | Online Info portal | A++ | Publications | Newspaper | B |
Publications | Online Info portal | A++ | Online Info portal | Newspaper | B |
College Visits | Online Info portal | A++ | College Visits | Newspaper | B |
Publications | Coaching Inst | A+ | Online Info portal | Publications | C |
Online Info portal | Coaching Inst | A+ | College Visits | Publications | C |
College Visits | Coaching Inst | A+ | Newspaper | Publications | C |
Newspaper | Coaching Inst | A+ | Edu Tours | Publications | C |
Edu Tours | Coaching Inst | A+ | Coaching Inst | Publications | C |
Newspaper | Edu Tours | A | Publications | College Visits | D |
Coaching Inst | Edu Tours | A | Online Info Port | College Visits | D |
Publications | Edu Tours | A | Newspaper | College Visits | D |
Online Info Port | Edu Tours | A | Edu Tours | College Visits | D |
College Visits | Edu Tours | A | Coaching Inst | College Visits | D |
[/fusion_table]
Step 4: Allocate resources, time and money according to the probability score –
So larger the probability of engagement when there is a result in the system, more should be the allocation of your money, resources (human, IT, etc), and time. These are directly proportional. You have, by now, already figured out the high engagement channels, the better combos, and also the dynamics of changing the calculations as per the life cycle of a candidate/consumer over a longer time period.
Step 5: Measure “demand generation”
If you have designed the most conducive marketing ecosystem that aids in continuous generation of demand from the market, and have invested into the channels as per the calculations above, then you are looking at strong and continuous revenue/result generation as well. Please note that as marketers, our jobs have gone beyond the traditional and we are now responsible for the success of a business or an organization, while improving the lives of our consumers. Understand the “demand generation” for your brand across these multiple channels, benchmark them against the other players, and scalably improve it over the time period to achieve business success for your organizations.
I would love to hear your feedback and comments on this model and the way this may help you develop better marketing systems at your end. If there is anything you feel can be added to this, then it would be great to have your comments on the same and we can discuss further.